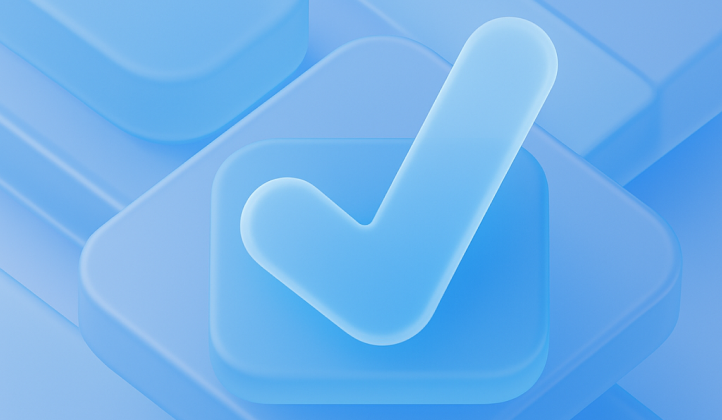
AI for Environmental Monitoring: Transforming Compliance and Control in Pharma
Discover how AI improves environmental monitoring, driving sustainability and valuable insights. Read the article to explore its transformative impact.
In pharmaceutical manufacturing, the margin for error is razor-thin. Environmental Monitoring (EM) has long been the first line of defense in ensuring aseptic conditions, cleanroom integrity, and regulatory compliance. But as regulatory scrutiny increases and the complexity of cleanroom operations grows, traditional methods of EM are showing their limitations.
In this blog, we explore how AI is reshaping environmental monitoring in pharma—making it not only faster and smarter, but also more predictive and compliant. AI’s ability to analyze and process large datasets related to ecological indicators, such as environmental data, is revolutionizing the field. For leaders tasked with scaling quality without scaling headcount, this shift isn’t just a trend. It’s a strategic transformation. Addressing challenges related to the implementation of AI technologies for environmental monitoring, such as data privacy, model bias, and ensuring data quality, is crucial for maximizing the effectiveness of AI solutions in addressing environmental issues. AI-driven monitoring systems enhance predictive accuracy and enable timely responses to ecological disturbances, further promoting sustainability and effective management of natural resources. Additionally, addressing air and water pollution as part of AI's role in environmental monitoring is significant for improving residents' quality of life and achieving the United Nations Sustainable Development Goals (SDGs).
From Reactive to Predictive: AI’s Role in Modern Environmental Monitoring
Traditional EM relies heavily on scheduled sampling, manual documentation, and retrospective investigation. By contrast, AI-driven systems:
- Analyze particle and microbial trends in real time
- Utilize predictive analytics to forecast environmental trends and predict excursions before they occur, with climate modeling as a critical tool for forecasting environmental trends
- Leverage historical data to improve predictive models and automatically adjust sampling plans based on risk profiles. AI algorithms are capable of identifying patterns in environmental data to enhance predictive accuracy.
The result? Environmental control becomes proactive rather than reactive—an outcome regulators increasingly expect under modern GMPs.
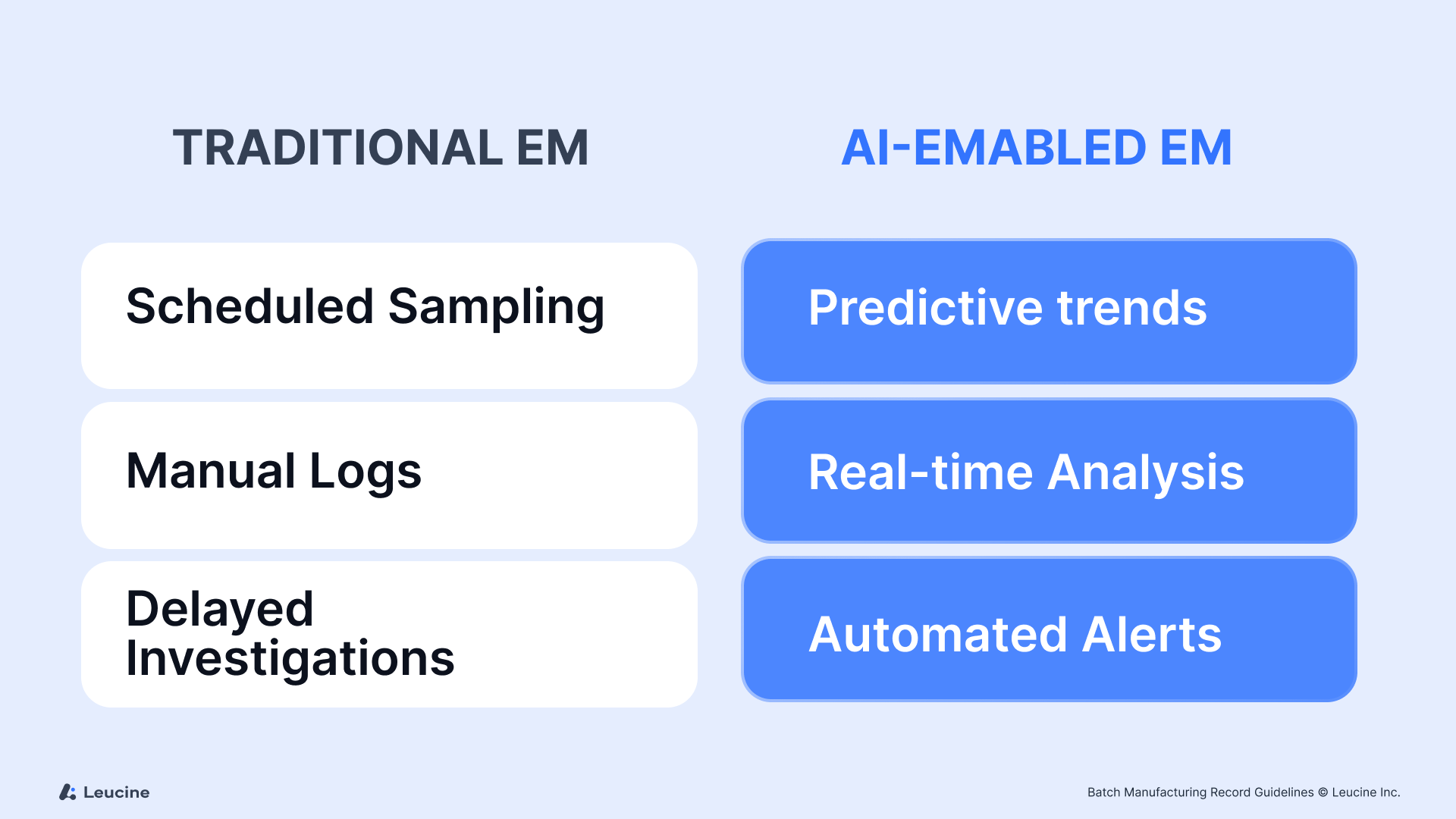
Applications of AI in Environmental Monitoring Workflows
AI doesn’t replace the fundamentals of viable and non-viable monitoring—it enhances them by identifying trends in vast amounts of climate and environmental data. Here’s how:
- Automating data collection and analysis by processing vast datasets
- Predicting environmental changes
- Enhancing real-time monitoring
- Supporting decision-making processes
- Developing early warning systems to predict extreme weather events and hazardous material releases
AI also leverages satellite imagery to monitor environmental changes, such as deforestation and habitat alterations, aiding in conservation efforts and informed decision-making.
1. Anomaly Detection in Particle Counts Using Machine Learning Algorithms
Machine learning models can establish cleanroom-specific baselines and detect deviations in real time by identifying patterns in particle counts using machine learning algorithms, flagging patterns that human analysts might miss.
2. Microbial Risk Scoring
AI tools can classify microbial isolates, identify pollution sources, and assign contamination risk scores based on frequency, source, and recurrence. These tools also enhance pollution monitoring by integrating real-time data collection and analysis from various environmental sensors.
Promoting sustainable practices in cleanroom environments is essential for reducing contamination and maintaining cleanroom integrity. Additionally, AI plays a crucial role in addressing environmental pollution through real-time analysis and response strategies, helping to mitigate the detrimental impact on health and ecosystems.
3. Smart Alert/Action Level Management
Instead of fixed thresholds, AI can suggest dynamic limits based on time, process phase, or operator activity—providing context-aware alerting and ensuring environmental compliance by adhering to regulatory standards.
Additionally, AI-driven monitoring systems play a crucial role in real-time tracking and management of air and water quality. These sophisticated systems employ sensors and machine learning algorithms to detect pollutants, provide immediate feedback to authorities, and facilitate quick responses to environmental threats, emphasizing their importance in effective pollution control and compliance with regulations. Air quality monitoring, in particular, benefits from the integration of AI and IoT, enabling predictive capabilities that are essential for public health and environmental safety.
Moreover, AI can help reduce carbon emissions through optimized decision-making processes. By leveraging reinforcement learning, AI systems can enhance resource allocation, minimize emissions, and adapt to dynamic climate conditions, thereby advancing overall climate resilience strategies.
4. Digital Investigation Assistance
When excursions occur, AI can suggest likely root causes by correlating environmental and ecological data from HVAC logs, personnel movement, and past events.
AI models enhance predictive capabilities and investigation accuracy by analyzing complex datasets, including satellite images, improving ecosystem health assessments, and optimizing agricultural practices.

📘 Want to understand the foundations before diving into AI? Read the Complete Guide to Environmental Monitoring in Pharma to learn cleanroom classifications, viable vs. non-viable sampling, alert levels, and more.
Business Impact for Pharma Leaders
For CEOs, CXOs, and QA Heads, AI in EM delivers benefits that go beyond technical improvements:
- Audit Readiness: Investigations become data-driven, transparent, and fast, providing crucial insights for climate resilience and addressing climate change. Understanding environmental processes is essential for developing effective AI models that can accurately simulate climate phenomena and enhance predictive capabilities.
- Resource Optimization: Fewer manual reviews, faster decisions, higher throughput. Sustainable management of natural resources is essential in the pharmaceutical industry to ensure long-term viability and compliance with environmental regulations.
- Compliance Confidence: Intelligent SOP triggers and historical trend justification.
AI reduces the lag between detection and action, which is especially critical when releasing batches or responding to inspections. Additionally, AI improves pollution monitoring and response strategies, significantly impacting human health by mitigating risks associated with respiratory diseases, cardiovascular issues, and cancer.
Environmental Monitoring Regulatory Alignment
While regulators don’t mandate AI, their guidance clearly supports:
- Data-driven risk assessment (EU Annex 1)
- Continuous environmental control (FDA Aseptic Processing Guidance)
- Trend-based alert level management (USP < 1116>)
- Enhancing compliance with environmental regulations through advanced data analysis and predictive modeling
AI also addresses various environmental challenges by offering innovative solutions for real-time data analysis and proactive responses. This includes tracking greenhouse gas emissions, which is crucial for regulatory compliance and developing strategies to mitigate climate change.
In fact, AI makes it easier to meet and exceed these expectations by providing documented evidence of ongoing process control. Additionally, AI plays a crucial role in environmental compliance by ensuring adherence to environmental laws through improved monitoring, reporting, and data analysis.
Why AI-Enabled EM is the Future
The pharmaceutical industry is entering a phase where digital maturity will directly correlate with audit outcomes, contributing to a sustainable future by leveraging AI for long-term sustainability. Facilities using AI for EM will:
- Have shorter deviation resolution cycles
- Identify contamination sources faster
- Minimize batch rejections by utilizing predictive models to forecast potential issues such as sea level rise and enhance compliance
AI can also analyze climate patterns to enhance predictive models, providing valuable insights for environmental monitoring.
These aren’t future predictions. They’re outcomes being realized today by leaders who are digitizing their operations.

Leverage AI with Leucine’s Environmental Monitoring Software
Leucine’s Environmental Monitoring platform incorporates AI systems to:
- Trend microbial and particulate data in real time
- Highlight systemic risks before they escalate
- Automatically trigger investigations and CAPA workflows
- Align your cleanroom control program with Annex 1
- Utilize remote sensing technologies for comprehensive data collection
Environmental monitoring artificial intelligence plays a crucial role in improving predictive accuracy and ensuring compliance by leveraging real-time data to monitor environmental indicators effectively. Additionally, the software contributes to environmental sustainability by enhancing monitoring and compliance, thus promoting proactive measures to address ecological challenges.
🚀 Ready to upgrade your EM program with AI? Book a personalized demo of Leucine today →
Conclusion:
AI in environmental monitoring isn’t about replacing people. It’s about empowering teams with better insights, faster response, and predictive control. Pharma leaders who embrace this shift are not only future-proofing their compliance strategy—they’re gaining a decisive edge in operational excellence and product integrity.
If you’re still using static spreadsheets and manual logs, the future is already leaving you behind.